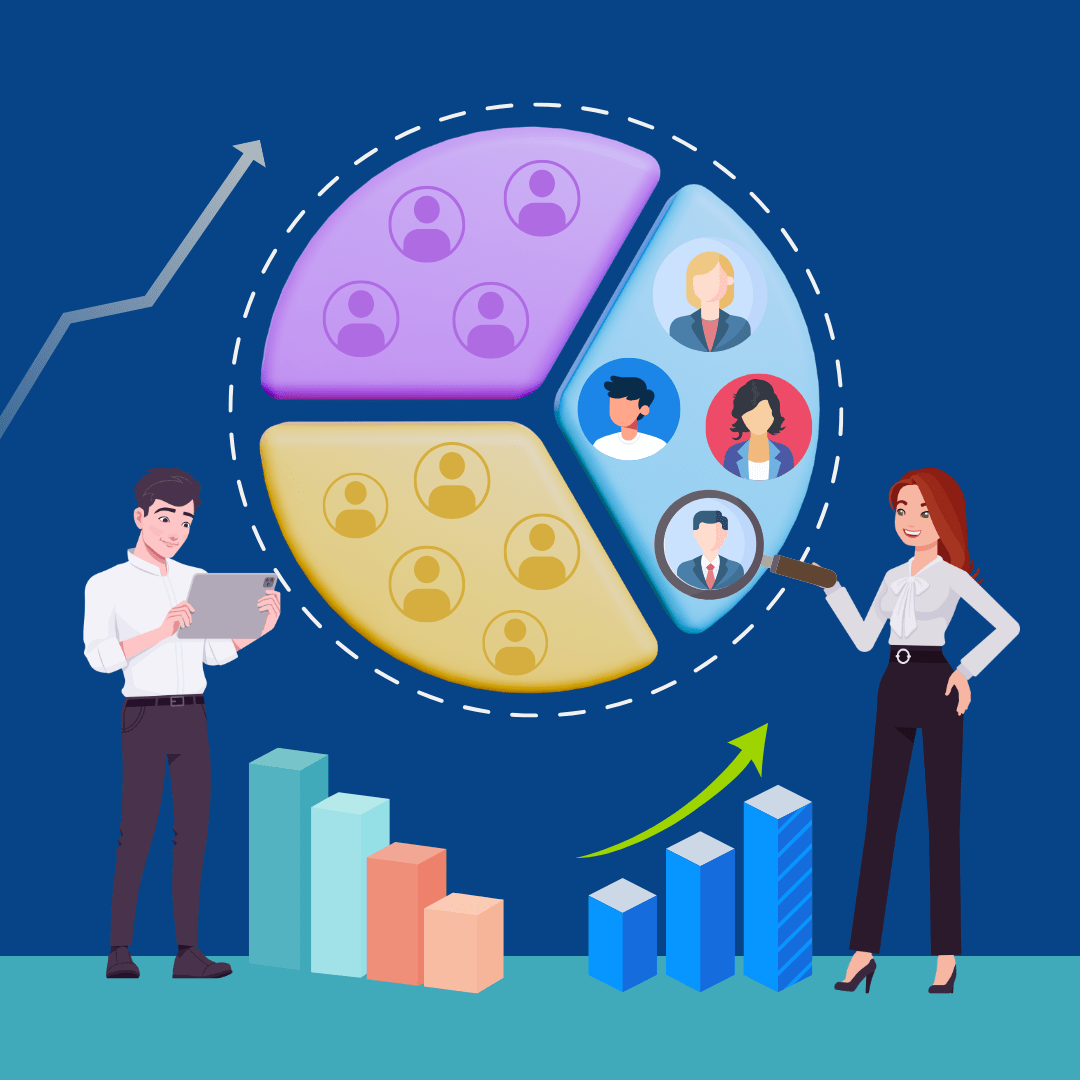
Probability sampling is a foundational method in statistics used to create accurate, representative samples from larger populations. By providing each member of a population with a known chance of selection, probability sampling reduces bias and increases the reliability of research outcomes. This guide explores the different types, methods, and practical uses of probability sampling and offers clear examples to help you understand how it works.
What is Probability Sampling?
Probability sampling is a sampling technique where each member of a population has a calculable chance of being selected for a study. This method ensures that the sample represents the population accurately, reducing sampling bias and making statistical conclusions more reliable.
In research and surveys, probability sampling is crucial for making valid inferences about large populations based on the responses from a smaller, manageable sample.
Why is Probability Sampling Important?
Probability sampling is essential because it ensures:
- Representativeness: Provides a sample that mirrors the entire population, making study outcomes more applicable.
- Reduced Bias: With calculated selection, each member has an equal opportunity of being chosen, minimizing bias.
- Accurate Predictions: Results obtained from probability samples can be more confidently generalized to the broader population.
Types of Probability Sampling
1. Simple Random Sampling
In simple random sampling, every individual in the population has an equal chance of being selected. This type is often chosen when the population is small and homogenous, as it requires no complex division or grouping.
- Example: Suppose you want to study students’ preferences for online courses at a small college. By assigning each student a number and using a random number generator, you can randomly select students to ensure each has an equal probability of selection.
2. Systematic Sampling
Systematic sampling involves selecting samples based on a fixed interval. The researcher first randomly selects a starting point and then picks every nth element in the population.
- Example: In a factory, if you want to test product quality, you might inspect every 20th item on the production line to ensure uniform quality control.
3. Stratified Sampling
Stratified sampling divides the population into different subgroups or “strata” based on shared characteristics, such as age, gender, or education level. Researchers then randomly sample from each stratum to ensure representation across all groups.
- Example: For a survey on work satisfaction across various departments in a company, stratified sampling ensures you get responses from each department, representing the diversity of opinions.
4. Cluster Sampling
In cluster sampling, researchers divide the population into clusters, usually based on geographic locations, and then randomly select entire clusters to include in the study. This method is helpful when dealing with large, geographically dispersed populations.
- Example: To study community health behaviors across a country, researchers might randomly select specific cities (clusters) to include, simplifying the sampling process.
5. Multistage Sampling
Multistage sampling combines different sampling techniques to gather data from larger populations efficiently. Researchers may start with cluster sampling and then apply random or systematic sampling within selected clusters.
- Example: A national educational study could first divide schools by region (clusters) and then randomly select students within each selected school, giving a comprehensive snapshot of the nation’s student body.
Methods of Probability Sampling
Probability sampling can use various methods to ensure every individual has an equal chance of selection. These methods are often applied based on the study’s goals and the population size.
- Random Number Generation: Uses software or tools to randomly select individuals in simple random sampling.
- Sampling Frame Creation: Builds an accurate list of the population to ensure everyone is accounted for in the sampling process.
- Use of Intervals: Systematic sampling relies on selecting intervals, such as every 10th item, to provide regular selection across the population.
- Stratification of Data: Ensures diverse characteristics, such as age or gender, are considered in stratified sampling to maintain population representation.
Examples of Probability Sampling in Real-Life Research
- Political Polling: Election surveys often use stratified sampling to ensure representation across demographic groups like age, race, and location, predicting election outcomes with higher accuracy.
- Market Research: Companies might use cluster sampling to gauge product preferences by region, obtaining insights on consumer preferences without sampling the entire country.
- Public Health Surveys: For national health surveys, researchers use multistage sampling, often beginning with regional clusters, then selecting individuals within those areas to estimate nationwide health trends.
Advantages of Probability Sampling
- Reduces Bias: Every member has an equal or known chance of selection.
- High Representativeness: Provides accurate representation, especially valuable in large populations.
- Reliability and Validity: Enhances the trustworthiness of study conclusions, as probability sampling supports reliable generalization to the broader population.
- Versatility: With different types and methods, probability sampling is adaptable for diverse research needs, from small local studies to large-scale national surveys.
Disadvantages of Probability Sampling
- Time-Consuming: Gathering a complete sampling frame and implementing randomization can require significant time and resources.
- Complexity in Large Populations: Applying methods like stratified or cluster sampling in large, diverse populations can be complicated.
- Cost: The meticulous processes required for probability sampling often result in higher costs compared to non-probability sampling methods.
When to Use Probability Sampling?
Probability sampling is most suitable when:
- Accuracy is Crucial: Studies that aim to make generalizable predictions about the population.
- Large Populations: Ideal for large or geographically spread populations.
- Diversity of Responses is Needed: When subgroups in a population must be represented to understand different perspectives, as in public policy surveys.
Probability Sampling vs. Non-Probability Sampling
Probability Sampling | Non-Probability Sampling |
Each member has a known chance of selection | Not all members have a known chance of being selected |
Reduces sampling bias | Higher risk of sampling bias |
High reliability for statistical analysis | Limited generalizability |
Costly and time-intensive | More cost-effective and quicker |
Conclusion
Probability sampling is a powerful tool in research, offering methods to accurately represent populations, reduce bias, and increase reliability. Understanding the types—simple random, systematic, stratified, cluster, and multistage—and their applications helps researchers design effective studies and draw meaningful, generalizable conclusions. By choosing the right probability sampling technique, researchers can confidently rely on their data to reflect true population characteristics.
Q1: What is the primary benefit of probability sampling?
A: Probability sampling provides a representative sample of the population, ensuring reliable and unbiased study results.
Q2: Can probability sampling be used for small populations?
A: Yes, probability sampling can be used for small populations, although it is often more valuable for larger populations where representativeness is critical.
Q3: How does probability sampling differ from non-probability sampling?
A: Probability sampling allows every member a known chance of selection, reducing bias, while non-probability sampling does not guarantee equal chances, increasing the risk of bias.
Q4: What is the most common type of probability sampling?
A: Simple random sampling is one of the most common types, as it is straightforward and ensures equal selection chances.
Q5: Why is stratified sampling used?
A: Stratified sampling ensures all key subgroups within a population are represented, making it valuable when different segments must be studied individually.
Explore More: