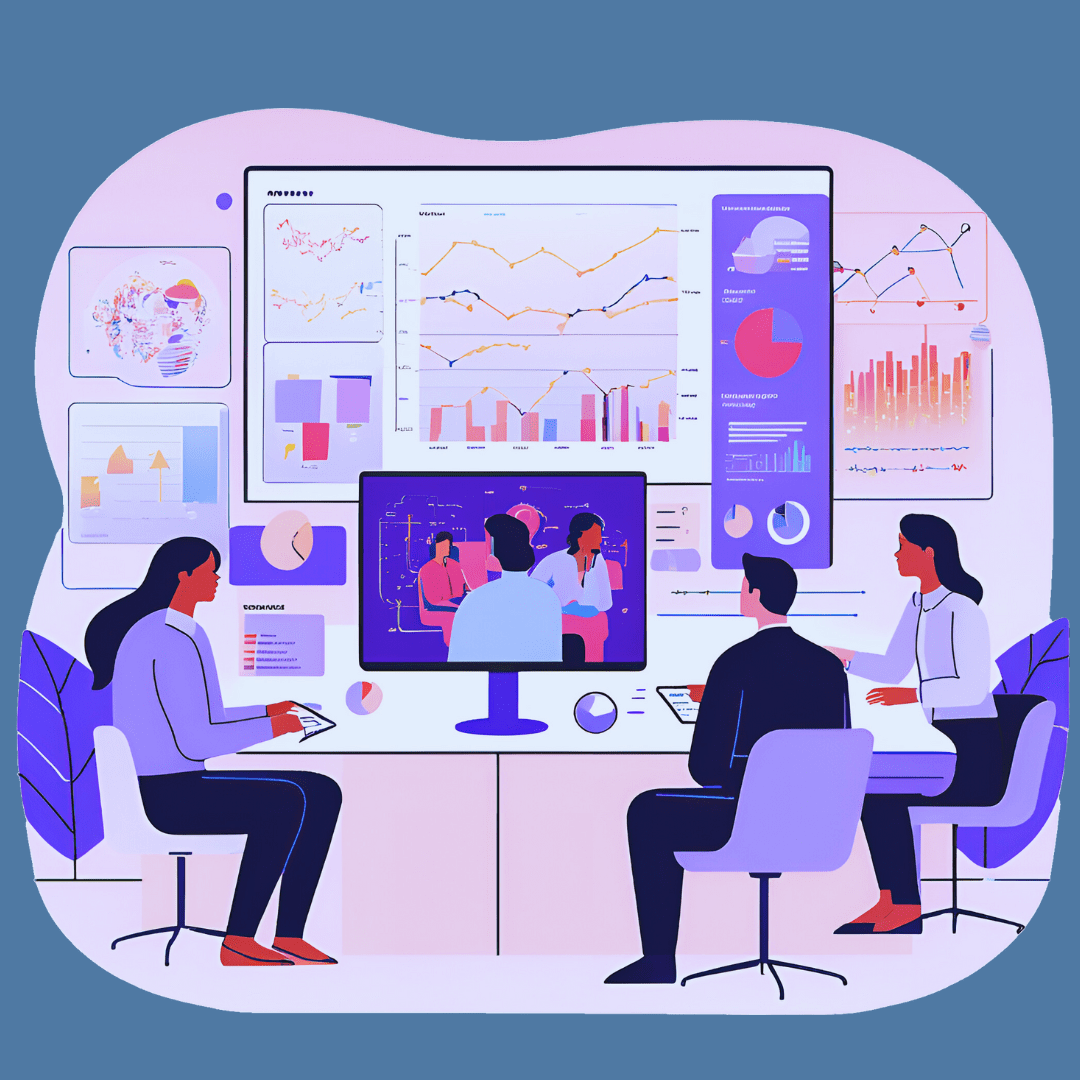
In today’s fast-paced business world, accurately predicting market trends can give companies a significant competitive advantage. Predictive analytics, which leverages historical data, statistical algorithms, and machine learning, enables businesses to forecast market trends with a high degree of accuracy. This data-driven approach allows companies to anticipate shifts in consumer behavior, identify potential opportunities, and make informed strategic decisions.
In this article, we’ll explore how predictive analytics works in market forecasting, the benefits it offers, and how businesses can use it to stay ahead of the curve.
What is Predictive Analytics?
Predictive analytics involves using data to make predictions about future outcomes. By analyzing historical data and applying algorithms, predictive analytics identifies patterns that can help forecast future trends. This process relies on a combination of data mining, machine learning, and statistical modeling to provide actionable insights, making it one of the most valuable tools for today’s market researchers and strategists.
In market forecasting, predictive analytics helps companies estimate demand, anticipate consumer preferences, and optimize business strategies by providing insights into likely future developments.
Why Predictive Analytics is Essential for Market Forecasting
With predictive analytics, businesses can move beyond traditional market research methods, which often rely on descriptive data, to a forward-looking approach. Here’s why predictive analytics is essential for effective market forecasting:
- Enhanced Accuracy in Forecasting
Traditional forecasting often relies on assumptions that can lead to inaccuracies. Predictive analytics, however, bases its predictions on large datasets and advanced algorithms, allowing for more precise forecasts. This accuracy helps businesses minimize risks by planning for specific scenarios. - Improved Decision-Making
By identifying potential future trends and outcomes, predictive analytics helps decision-makers make more informed choices. Knowing what might happen allows companies to respond proactively, whether by adjusting pricing strategies, launching new products, or repositioning their brand. - Increased Agility and Competitive Advantage
Predictive analytics helps companies stay agile by anticipating shifts in the market before they happen. This ability to adapt quickly to changing trends offers a significant competitive advantage, enabling companies to capture market share ahead of competitors. - Personalized Customer Experiences
By predicting individual consumer behavior, businesses can personalize their marketing strategies. Predictive analytics helps companies understand consumer preferences and behaviors, allowing them to create tailored messages, offers, and experiences.
Key Components of Predictive Analytics in Market Forecasting
To effectively use predictive analytics in market forecasting, it’s important to understand its core components and techniques:
1. Data Collection
Predictive analytics relies on high-quality data, including historical sales data, customer demographics, website activity, and even social media engagement. Collecting relevant data from multiple sources provides a solid foundation for accurate forecasting.
2. Data Preprocessing and Cleaning
Raw data often contains errors, outliers, or inconsistencies that can impact predictive accuracy. Data preprocessing involves cleaning, transforming, and organizing data to ensure it’s accurate, consistent, and ready for analysis.
3. Predictive Modeling
Predictive models use algorithms to analyze patterns and relationships in historical data. Popular models include:
- Regression Analysis: Estimates the relationships between variables, useful for predicting continuous outcomes such as sales volume.
- Decision Trees: A flowchart-like structure used to make decisions and predictions by splitting data into branches based on certain conditions.
- Neural Networks: Mimics human brain functions to identify complex patterns, especially useful for analyzing unstructured data like text or images.
- Time Series Analysis: Focuses on data points collected over time, ideal for tracking and forecasting seasonal trends or sales patterns.
4. Model Training and Testing
Predictive models need to be trained on historical data to learn and make accurate predictions. After training, the model is tested on a separate data set to validate its accuracy. This step helps fine-tune the model and ensures its predictions align with real-world data.
5. Ongoing Model Optimization
Predictive models need regular updates as new data becomes available. This continuous optimization improves prediction accuracy over time and ensures the model remains relevant as market dynamics change.
Applications of Predictive Analytics in Market Forecasting
Predictive analytics can be applied across various areas of market forecasting to improve strategic planning and operational efficiency:
1. Demand Forecasting
One of the primary uses of predictive analytics is estimating future demand for products or services. By analyzing factors such as historical sales, seasonal patterns, and economic indicators, businesses can forecast demand with higher accuracy, optimizing inventory levels and reducing stockouts or overstock.
2. Customer Behavior Prediction
Predictive analytics helps brands understand what drives consumer behavior, from purchasing patterns to churn risk. With these insights, companies can create targeted marketing strategies, reduce customer churn, and increase loyalty by addressing customer needs proactively.
3. Pricing Optimization
Predictive analytics enables dynamic pricing strategies by forecasting how consumers will respond to different pricing levels. By analyzing competitor prices, customer preferences, and demand elasticity, companies can set optimal prices that maximize revenue while remaining competitive.
4. Market Segmentation and Targeting
Predictive analytics helps companies identify and understand different customer segments, allowing for more precise targeting. By forecasting which demographics are more likely to respond to certain products or promotions, companies can focus their resources on high-potential segments, improving marketing ROI.
5. Product Development
Predictive analytics allows companies to assess potential demand for new products or features by analyzing trends and customer feedback. Companies can prioritize products that align with predicted market needs, reducing the risk associated with new launches.
Benefits of Predictive Analytics in Market Forecasting
Adopting predictive analytics provides a host of advantages, from better resource allocation to enhanced customer satisfaction:
- Reduced Operational Costs: Accurate forecasting helps businesses optimize inventory and allocate resources more effectively, reducing costs associated with excess stock or emergency restocking.
- Enhanced Customer Satisfaction: Predictive insights into customer behavior allow brands to meet consumer needs proactively, improving overall satisfaction and loyalty.
- Data-Driven Competitive Advantage: Predictive analytics provides a factual basis for strategic planning, enabling companies to stay ahead by adapting to changes in consumer preferences and market dynamics.
Challenges in Using Predictive Analytics for Market Forecasting
While predictive analytics offers significant benefits, it also comes with challenges that require careful consideration:
1. Data Quality Issues
Predictive analytics depends on high-quality data, but inconsistent or incomplete data can lead to inaccurate predictions. To mitigate this, companies should prioritize robust data collection processes and regular data validation.
2. Model Complexity and Maintenance
Predictive models can be complex and require ongoing optimization to remain accurate. This requires investment in both time and resources, including skilled data scientists who can manage and refine the models.
3. Privacy Concerns
With predictive analytics heavily reliant on customer data, privacy issues can arise. Companies must ensure compliance with data protection regulations, such as GDPR, CCPA and DPDPA, to protect consumer privacy and maintain trust.
4. Interpretation of Results
Predictive models can provide a wealth of data, but interpreting these results can be challenging. Businesses need skilled analysts who can translate predictions into actionable insights that drive decision-making.
Best Practices for Implementing Predictive Analytics in Market Forecasting
To maximize the effectiveness of predictive analytics in market forecasting, consider these best practices:
- Start with Clear Goals
Define specific objectives for predictive analytics to ensure the analysis aligns with business goals. Clear objectives streamline the model development process and improve focus. - Invest in High-Quality Data
Quality data is crucial for predictive accuracy. Implement data management practices that ensure data accuracy, consistency, and relevance. - Use a Combination of Models
Different predictive models excel in different scenarios. Combining multiple models—such as regression for sales forecasting and decision trees for customer segmentation—can improve accuracy. - Regularly Update and Validate Models
Market conditions change, and predictive models must adapt to stay accurate. Regular updates and validations ensure the model remains reliable and relevant over time. - Prioritize Data Privacy
Comply with all relevant data privacy regulations to protect customer information and avoid potential legal issues. Transparency in data usage builds consumer trust and supports long-term data availability.
Conclusion: Embracing Predictive Analytics for a Competitive Edge
Predictive analytics is a powerful tool that enables businesses to forecast market trends, enhance decision-making, and respond proactively to changing consumer behaviors. From demand forecasting to customer segmentation, predictive analytics offers invaluable insights that support strategic planning and improve operational efficiency. While challenges exist, such as data quality and model complexity, businesses that invest in predictive analytics and adopt best practices can gain a significant competitive advantage. As the market landscape continues to evolve, embracing predictive analytics will be essential for staying ahead and driving sustainable growth.
1. What is predictive analytics in market forecasting?
Predictive analytics uses historical data and algorithms to forecast future market trends, enabling businesses to anticipate demand and understand consumer behavior.
2. How does predictive analytics improve decision-making?
It provides data-driven insights, allowing businesses to make informed choices and respond proactively to anticipated market changes.
3. What are common predictive models used in forecasting?
Common models include regression analysis, decision trees, neural networks, and time series analysis, each suited to different types of data.
Explore More: