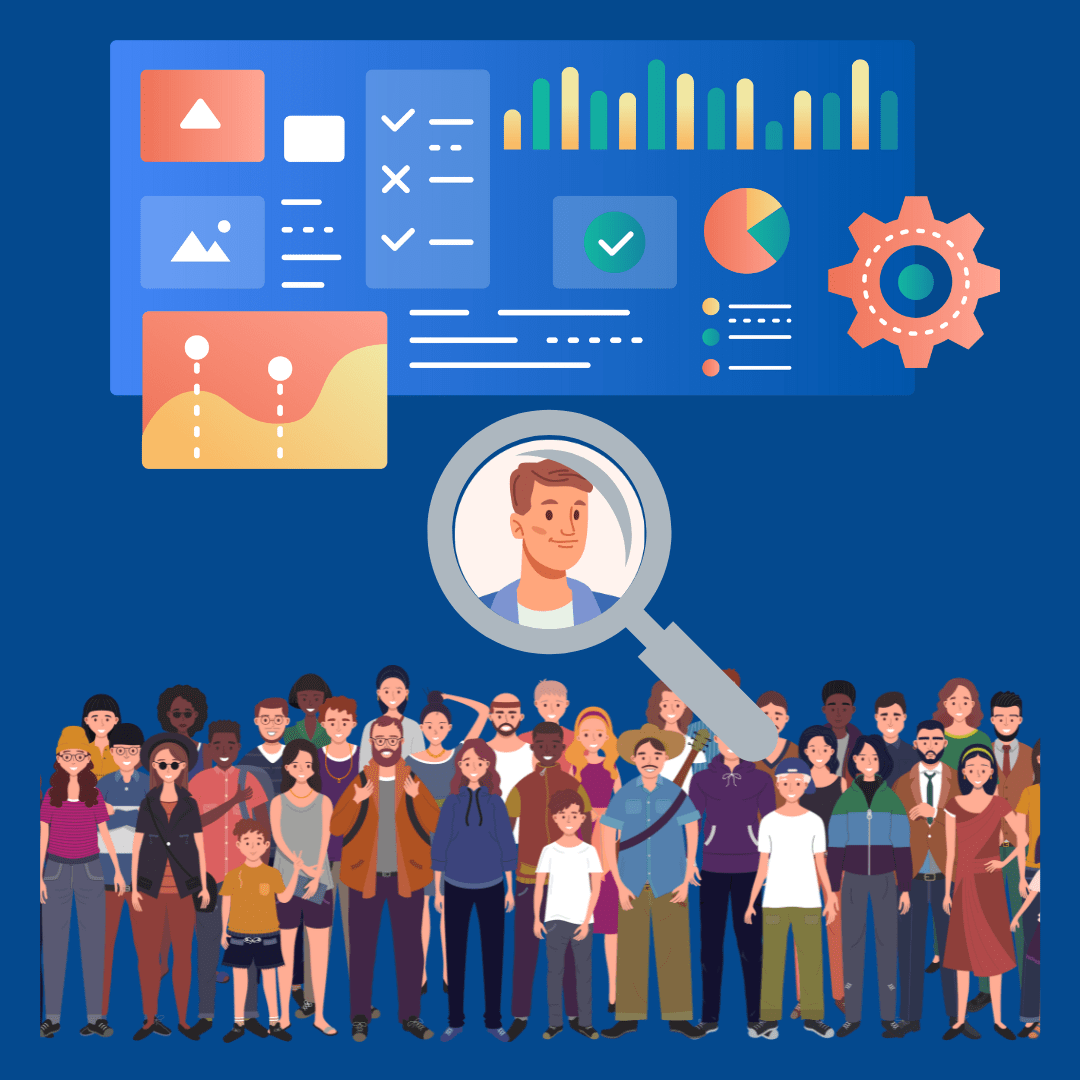
Sampling is a very basic notion in research, statistics, and many scientific fields. It only involves the process for selecting subsets of a given population, consisting either of individuals, items, or cases for making inferences about that population. The most noted role played by sampling in helping a researcher avoid this hassle of studying an entire population, which might be impracticable and time-consuming. This paper gives an overview of sampling, covering its importance, different sampling methods, and real applications.
What is Sampling?
Sampling may be defined as the selection of a certain number of subjects from the entire population or group. In any research work, the complete set of elements or subjects, in which the researcher is interested in, is called the population. Although in most situations it is impractical or impossible, because of limitations of time, money and access, to study the whole population of interest through a census. It offers a practical solution by the fact that sampling allows the analysis of only a portion of the population to generalize the findings.
Importance of Sampling
The importance of sampling in research cannot be overstated. It serves several critical functions:
- Efficiency: Sampling enables a quicker, more cost-effective method of collecting data than if it were based on studies of an entire population.
- Feasibility: In most cases, size or geographic distribution excludes the possibility of studying every individual in a population. Sampling makes research feasible.
- Accuracy: A good sample design may give very accurate parameter estimates of the population. The application of appropriate sampling methods will help minimize bias and error.
- Resource Management: Sampling allows for a much more effective and efficient use of resources, such as time, money, and people, due to the fact that research is conducted on smaller rather than large groups.
Types of Sampling Methods
Generally, there are two major types of sampling methods: probability sampling and non-probability sampling. Each group includes some particular techniques which use different research settings.
1. Probability Sampling
Probability sampling methods are those where each element of the population has a known, nonzero chance of selection. This is often preferred for generalization of results to the larger population in quantitative research.
a. Simple Random Sampling
The simplest will be simple random sampling, where every individual in the population has an equal chance of selection. This can be done by generating random numbers or lotteries. It works very well if the population is homogeneous.
b. Stratified Sampling
It involves dividing a population into subgroups, called strata, based on certain characteristics (like age, gender, etc.), and then drawing a random sample from these strata. This ensures that there will be a representation of all important subgroups in the sample and thus leads to greater precision in the results.
c. Systematic Sampling
Systematic sampling involves selecting every nth item in a population list. For instance, if you want to sample 100 out of 1,000, you may choose every 10th person. It is easy to conduct but requires that the population list be randomly ordered to avoid bias.
d. Cluster Sampling
This is employed when the population is geographically spread or organized into natural groups. In cluster sampling, as opposed to selecting individuals that are formed into groups on a geographical or other basis, whole clusters are randomly selected. All individuals within the chosen clusters are then studied. The technique is economical but may bring about sampling error if the chosen clusters are not representative.
2. Non-Probability Sampling
Non-probability sampling types do not permit every element of the population with an equal or known chance for selection. These types are typically employed in qualitative research or in scenarios where probability sampling is not possible.
a. Convenience Sampling
Convenience sampling means the researcher has sampled those individuals with whom it is convenient for them to interact. This method is easy to apply, fast, and does not involve any difficulties; however, it may lead to serious bias since it may fail to represent the population.
b. Purposive Sampling
Purposive sampling is when one goes ahead to choose the sample depending upon a given characteristic criterion that goes together with the research question. This method is widely used in qualitative research whereby key insights are sought after from a particular group of people.
c. Snowball Sampling
It is used in the case where the population to be sampled is difficult to reach or identify, such as in the instance of marginalized groups. This technique relies on earlier participants referring to other participants for a “snowball effect.” Besides, it comes in handy when one needs access to hidden populations, though it may not yield a representative sample.
d. Quota Sampling
Quota sampling is a technique in which a sample is selected that replicates the population’s characteristics in predetermined proportions. For example, if the population has 60% females, then the number of female subjects in the sample would be 60%. The advantage of this system is that it gives representation, but it may introduce bias if the selection itself is not random within these quotas.
Applications of Sampling
Sampling is used in a wide range of fields, from market research to public health, social sciences, and more. Here are a few key applications:
1. Market Research
In market research, sampling is used to gather insights into consumer behavior, preferences, and trends. Companies often use sampling to test new products, assess customer satisfaction, and forecast sales. By studying a representative sample of their target market, businesses can make informed decisions without needing to survey every customer.
2. Public Health
In public health, sampling is essential for conducting epidemiological studies, tracking disease outbreaks, and evaluating health interventions. For example, health surveys often rely on stratified sampling to ensure that various demographic groups are adequately represented, allowing for accurate assessments of public health needs.
3. Social Sciences
Social scientists use sampling to study human behavior, social trends, and cultural phenomena. Whether through surveys, interviews, or experiments, sampling allows researchers to gather data that is representative of larger populations, enabling them to draw meaningful conclusions and contribute to the development of theories.
4. Environmental Studies
In environmental studies, sampling is used to assess the quality of air, water, soil, and biodiversity. Environmental scientists often use cluster sampling to study different geographical areas, allowing them to monitor environmental changes and inform conservation efforts.
5. Quality Control
In manufacturing and quality control, sampling is used to inspect products and ensure they meet quality standards. For example, systematic sampling might be used on a production line to check every 50th item for defects. This approach helps identify and address quality issues without inspecting every single product.
Challenges in Sampling
While sampling is a powerful tool, it is not without challenges. Some common issues include:
- Sampling Bias: Occurs when the sample is not representative of the population, leading to skewed results. This can happen due to poor sampling design, non-random selection, or non-response bias.
- Sample Size: Determining the appropriate sample size is crucial. A sample that is too small may not provide reliable results, while a sample that is too large may be unnecessarily costly and time-consuming.
- Data Collection: Collecting data from the sample must be done carefully to avoid errors and ensure accuracy. Poor data collection methods can compromise the validity of the results.
Conclusion
Sampling is an intrinsic element of research, and often large populations are studied through manageable sub-sets which represent these populations. Proper sampling techniques will help a researcher derive data that are accurate and reliable in a cost-effective manner. Further, it aids in good decision-making in all fields. Even with the problems associated with sampling, it is still an important tool for executing effective research and insight into populations.
What is sampling in research?
Sampling refers to the process of selecting a subset of individuals or items from a larger population to make inferences about that population. It allows researchers to study a manageable portion of the population instead of the entire group.
Why is sampling important in research?
Sampling is important because it makes research more efficient, feasible, and cost-effective. It allows for accurate analysis with fewer resources, provides generalizable findings, and helps avoid the impracticalities of studying an entire population.
What is simple random sampling?
Simple random sampling is a probability method where every individual in the population has an equal chance of being selected. It’s often done using random number generators or lotteries.
What is stratified sampling?
Stratified sampling involves dividing the population into subgroups (strata) based on specific characteristics (e.g., age, gender) and then drawing random samples from each stratum to ensure representation of key subgroups.
What is convenience sampling?
Convenience sampling is a non-probability method where the sample is chosen based on ease of access and availability. While fast and simple, it may introduce bias as it may not represent the population.
What is cluster sampling?
Cluster sampling involves dividing the population into groups (clusters), usually based on geography or other criteria, and randomly selecting entire clusters for study. This method is cost-effective but may increase sampling error.
When is snowball sampling used?
Snowball sampling is used when the population is hard to reach or hidden, such as marginalized groups. In this method, existing participants refer new participants, creating a “snowball” effect.
What is sampling bias?
Sampling bias occurs when the sample does not accurately represent the population, leading to skewed results. It can arise from poor sampling design, non-random selection, or non-response from participants.
Explore More :