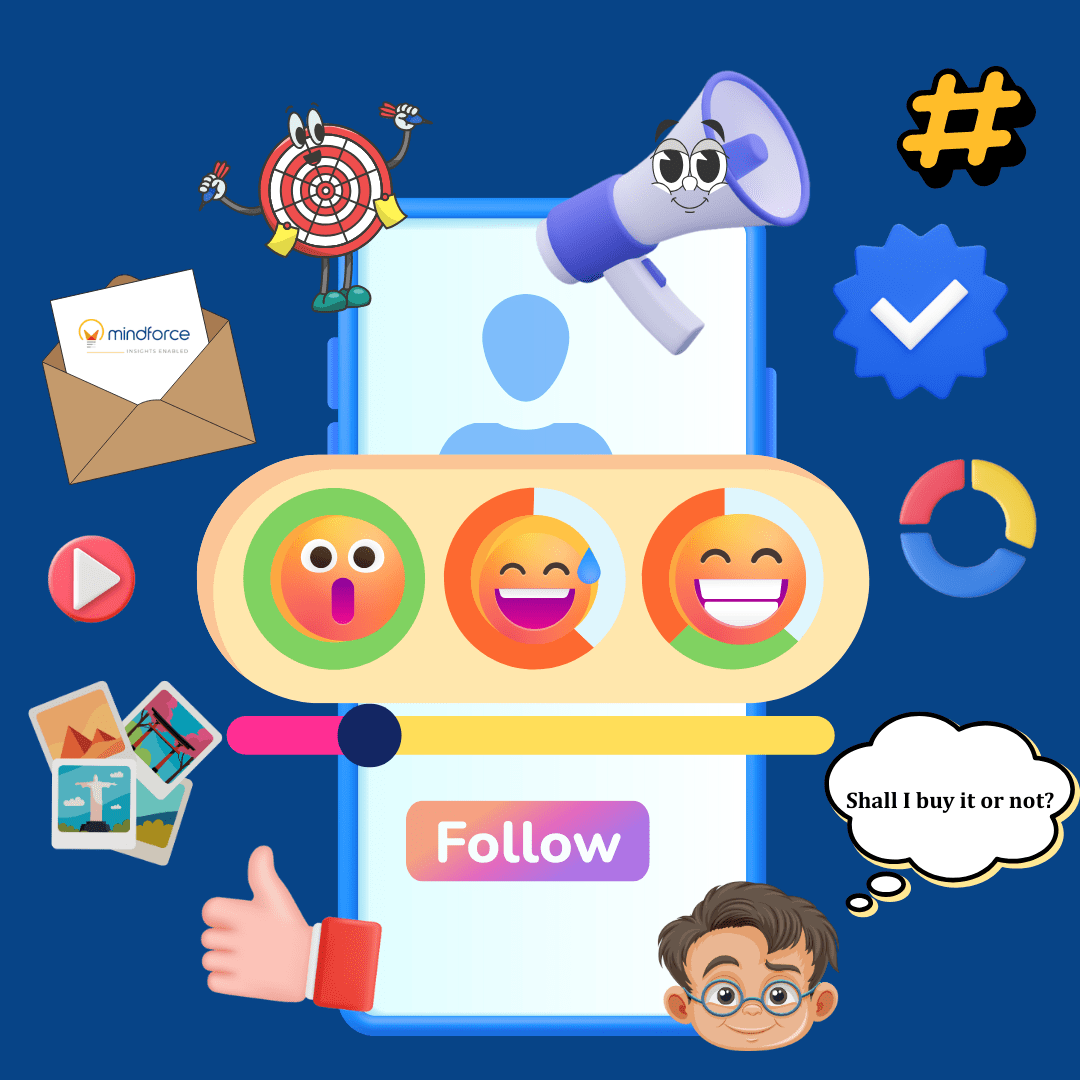
Introduction
In today’s digital landscape, understanding consumer emotions is vital for businesses aiming to improve their products, services, and marketing strategies. Sentiment analysis, also known as Opinion Mining, is a powerful tool that enables companies to analyze customer opinions and emotions through text, social media, reviews, and surveys. This data-driven approach provides valuable insights into consumer preferences and behaviors, allowing brands to tailor their strategies effectively.
This article explores the importance of sentiment analysis in market research, its methodologies, applications, and how businesses can leverage it to enhance customer engagement and brand perception.
What is Sentiment Analysis?
Sentiment analysis is the process of using natural language processing (NLP), artificial intelligence (AI), and machine learning (ML) to identify and categorize emotions expressed in textual data. It helps businesses gauge whether customer opinions are positive, negative, or neutral.
Types of Sentiment Analysis
- Polarity-Based Sentiment Analysis: Categorizes sentiments as positive, negative, or neutral.
- Emotion Detection: Identifies emotions such as happiness, anger, sadness, or frustration.
- Aspect-Based Sentiment Analysis: Focuses on specific aspects of a product or service.
- Intent Analysis: Determines whether a customer intends to buy, complain, or inquire.
Importance of Sentiment Analysis in Market Research
a) Enhancing Customer Experience
By analyzing customer feedback, businesses can identify pain points and areas for improvement, leading to enhanced customer satisfaction.
b) Tracking Brand Reputation
Sentiment analysis helps companies monitor online discussions about their brand, detecting potential PR crises before they escalate.
c) Competitive Analysis
Understanding how consumers perceive competitors allows businesses to refine their strategies and gain a competitive edge.
d) Data-Driven Decision Making
Companies can make informed marketing and product development decisions based on real-time consumer sentiment insights.
How Sentiment Analysis Works
a) Data Collection
The process begins with gathering textual data from sources like social media, online reviews, customer surveys, and blogs.
b) Data Processing
- Preprocessing: Cleaning data by removing irrelevant text, stop-words, and special characters.
- Tokenization: Breaking text into individual words or phrases.
- Stemming and Lemmatization: Standardizing words to their base forms.
c) Sentiment Classification
Using AI models and lexicons, sentiment is classified into positive, negative, or neutral categories. Machine learning models such as Naive Bayes, LSTM, and BERT enhance accuracy.
d) Interpretation & Insights
Once sentiment is analyzed, businesses can extract valuable insights to understand customer emotions and trends.
Tools and Technologies for Sentiment Analysis
a) AI-Powered Sentiment Analysis Tools
- IBM Watson: AI-driven sentiment analysis with NLP capabilities.
- Google Cloud Natural Language API: Advanced text analysis and sentiment detection.
- Lexalytics: Customizable sentiment analysis for business intelligence.
b) Social Media Sentiment Analysis Tools
- Hootsuite: Monitors social media sentiment and brand mentions.
- Brandwatch: Tracks consumer sentiment across digital channels.
- Sprout Social: Provides insights into social media audience sentiment.
Challenges in Sentiment Analysis
a) Sarcasm and Irony
Sentiment analysis tools may struggle with detecting sarcasm, leading to inaccurate interpretations.
b) Context Understanding
Language nuances, cultural differences, and ambiguous expressions can affect sentiment analysis accuracy.
c) Data Bias
If training data is biased, the sentiment analysis model may produce skewed results, impacting decision-making.
d) Evolving Language
Slang, abbreviations, and emojis constantly evolve, requiring AI models to adapt continuously.
Future Trends in Sentiment Analysis
a) Emotion AI & Advanced NLP
The integration of AI-powered emotion detection will enhance sentiment analysis accuracy.
b) Multilingual Sentiment Analysis
Expanding sentiment analysis capabilities across multiple languages will improve global consumer insights.
c) Voice Sentiment Analysis
Advancements in AI-driven voice recognition will allow sentiment analysis of audio interactions.
d) Real-Time Sentiment Monitoring
Businesses will increasingly use real-time sentiment tracking to respond proactively to consumer concerns.
Conclusion
Sentiment analysis is revolutionizing market research by providing deeper insights into consumer emotions and preferences. Businesses that leverage this technology effectively can enhance customer experiences, improve brand reputation, and make data-driven decisions to stay ahead in a competitive market. As AI and NLP technologies continue to evolve, sentiment analysis will become even more accurate and indispensable in understanding consumer behavior.
By adopting sentiment analysis, companies can move beyond traditional market research methods and embrace a more dynamic, customer-centric approach to business strategy.
1. What is sentiment analysis in market research?
Sentiment analysis is the use of AI and NLP to analyze consumer opinions and emotions in text data, such as social media, reviews, and surveys.
2. Why is sentiment analysis important for businesses?
It helps businesses improve customer experience, monitor brand reputation, conduct competitive analysis, and make data-driven decisions.
3. What are the different types of sentiment analysis?
The key types include polarity-based analysis, emotion detection, aspect-based analysis, and intent analysis.
4. How do businesses collect data for sentiment analysis?
Companies gather data from online reviews, social media platforms, customer surveys, and blogs.
5. What tools are commonly used for sentiment analysis?
Popular tools include IBM Watson, Google Cloud Natural Language API, Hootsuite, Brandwatch, and Sprout Social.
6. What challenges does sentiment analysis face?
Challenges include detecting sarcasm, understanding language context, dealing with biased data, and keeping up with evolving slang and emojis.
Explore More: